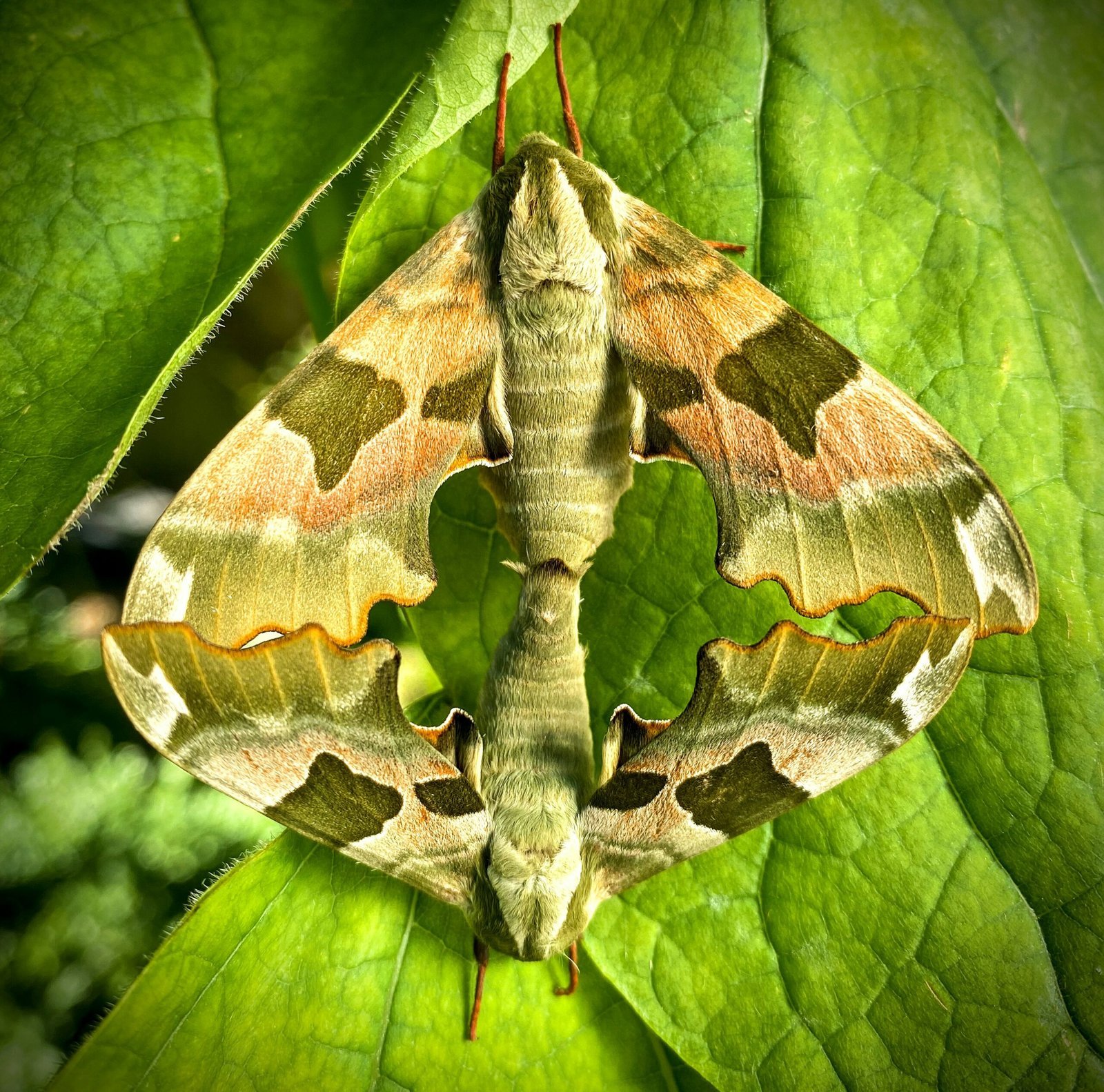
Introduction to Digital Twins in Transportation
The concept of digital twins has emerged as a groundbreaking innovation in the transportation sector. A digital twin is essentially a virtual replica of a physical asset, system, or process. It uses real-time data and advanced algorithms to create simulations that mirror the behavior and condition of its real-world counterpart. This technology leverages sensors, IoT devices, and artificial intelligence to provide a comprehensive and dynamic representation of physical entities.
Key components of a digital twin include data collection systems, analytic and simulation tools, and visualization platforms. These elements work together to gather data from the physical asset, process and analyze the information, and present it in an accessible and interactive format. This integration of hardware and software allows for continuous monitoring and real-time updates, making digital twins instrumental in decision-making and operational optimization.
Currently, digital twins are being applied in several facets of transportation. They are used to monitor and manage traffic flow in urban areas, optimize logistics and supply chain operations, and enhance the performance of public transportation systems. For example, digital twins can simulate and predict traffic patterns, enabling city planners to design more efficient road networks. In logistics, they can track vehicle conditions and route efficiency, leading to reduced fuel consumption and timely deliveries.
The benefits of integrating digital twins in transportation are manifold. Enhanced efficiency is one of the primary advantages, as digital twins can streamline operations and reduce downtime. Safety is another critical benefit; by continuously monitoring the condition of vehicles and infrastructure, potential issues can be identified and addressed proactively, thereby reducing the likelihood of accidents and failures. Moreover, digital twins facilitate predictive maintenance, allowing for timely interventions before equipment failure occurs, resulting in cost savings and increased reliability.
As this technology continues to evolve, its potential applications and benefits in transportation are expected to expand, paving the way for smarter, safer, and more efficient transportation systems worldwide.
Technological Advancements Driving Digital Twin Adoption
In recent years, significant technological advancements have spurred the widespread adoption of digital twins across the transportation sector. At the forefront of this transformation are Internet of Things (IoT) devices, which have become integral to the creation and operation of digital twins. IoT devices gather real-time data from various transportation system components, such as vehicles, infrastructure, and environmental sensors. This data is then used to create detailed and dynamic models of these systems, allowing for enhanced monitoring and optimization.
Artificial Intelligence (AI) and machine learning innovations are also pivotal in driving the adoption of digital twins. These technologies enable the analysis and interpretation of the massive data sets generated by IoT devices. Through sophisticated algorithms, AI and machine learning can identify patterns, predict potential issues, and recommend corrective actions. This predictive capability is invaluable for improving operational efficiency and reducing maintenance costs in transportation networks.
Big data analytics plays a crucial role in managing the vast amounts of information collected by IoT devices. By employing advanced analytics techniques, transportation authorities can gain actionable insights into system performance and user behavior. This capability facilitates better decision-making and support for infrastructure planning, traffic management, and safety enhancements. The integration of big data analytics with digital twins thus transforms raw data into valuable intelligence, driving smarter, more resilient transportation systems.
The advent of 5G networks has significantly enhanced connectivity, further accelerating digital twin adoption in transportation. With its high-speed, low-latency communication capabilities, 5G enables real-time data exchange and seamless interaction between digital twins and physical assets. This connectivity boosts the responsiveness and accuracy of digital twins, allowing for immediate adaptations and improvements to transportation operations.
Together, these technological advancements—IoT, AI and machine learning, big data analytics, and 5G—form the backbone of digital twins in transportation. They facilitate detailed modeling and real-time monitoring, which in turn enhances the efficiency, reliability, and sustainability of transportation systems.
Applications and Use Cases of Digital Twins in Transportation
In the transportation sector, digital twins are revolutionizing various aspects, from urban planning to real-time traffic management. By creating virtual replicas of physical assets and systems, digital twins enable enhanced analysis and decision-making. Their practical applications are diverse, impacting smart cities, infrastructure projects, and fleet management through numerous case studies.
One prominent application is in the development of smart cities. Digital twins are utilized to simulate city environments, testing the effects of new infrastructure, transport routes, and public service implementations before actual deployment. For instance, the city of Singapore has adopted digital twin technology to model urban environments, predict traffic patterns, and enhance the efficiency of public transit systems. This has led to reduced congestion and optimized maintenance schedules, ultimately improving the quality of urban living.
Another significant use case is in real-time traffic management systems. Digital twins can monitor and analyze live data from various sensors and cameras across the road network. In Stockholm, Sweden, a digital twin system helps manage traffic flow by providing real-time data insights, enabling authorities to swiftly address congestion issues and reroute traffic. This application has led to substantial reductions in traffic delays and increased commuter satisfaction.
The technology also finds application in virtual simulation for infrastructure projects. Before constructing bridges, roads, or tunnels, engineers can use digital twins to simulate different scenarios, stress tests, and environmental impacts. London’s Crossrail project exemplifies this, where a digital twin was employed to anticipate construction challenges, resulting in significant cost savings and minimized project delays.
Furthermore, digital twins are enhancing fleet management in logistics. By creating digital replicas of vehicles, companies can monitor the condition, performance, and route efficiency of their fleets in real time. A notable example is DHL, which uses digital twins to optimize delivery routes, predict maintenance needs, and improve fuel efficiency. This has led to lower operational costs and improved service reliability.
Challenges and Future Outlook
The implementation and scaling of digital twins in the transportation sector present a host of challenges that must be carefully navigated. Chief among these concerns are data privacy and security. As digital twins rely heavily on real-time data collection, the risk of data breaches and unauthorized access to sensitive information is elevated. To mitigate these risks, stringent cybersecurity measures and robust encryption protocols must be employed. Additionally, adherence to regulatory standards and continuous monitoring for vulnerabilities are crucial.
Another significant challenge is the high initial investment cost required for the adoption of digital twins. These costs encompass the development and integration of sophisticated sensors, advanced analytical tools, and comprehensive training programs for personnel. This financial barrier can be particularly daunting for smaller transportation entities and may slow widespread adoption. Public-private partnerships, along with government grants and subsidies, could play a pivotal role in alleviating these financial constraints.
Interoperability between different systems and standards also poses a considerable hurdle. Transportation systems are often multifaceted and involve various stakeholders, each with their own proprietary technologies and protocols. Achieving seamless integration and data exchange across these disparate systems requires the establishment of universal standards and collaborative frameworks. Industry-wide initiatives and consortiums can facilitate the development of such standards, fostering a more cohesive digital ecosystem.
Looking ahead, the future of digital twins in transportation appears promising, driven by ongoing advancements in technology and supportive regulatory landscapes. Emerging technologies such as artificial intelligence and machine learning are poised to enhance the predictive capabilities and efficiency of digital twins. Furthermore, the adoption of 5G networks will enable faster data transmission, significantly improving the real-time responsiveness of these systems.
Regulatory bodies are increasingly recognizing the potential of digital twins and are beginning to adapt policies to support their deployment. By setting clear guidelines and creating favorable conditions for innovation, regulators can help accelerate the integration of digital twins into mainstream transportation practices.
As the industry embraces these advancements, digital twins are likely to become more accessible and widely adopted. This transformation holds the potential to revolutionize the transportation sector, enabling more efficient operations, predictive maintenance, and enhanced passenger experiences. Ultimately, the integration of digital twins could lead to smarter, safer, and more sustainable transportation networks, ushering in a new era of connectivity and innovation.