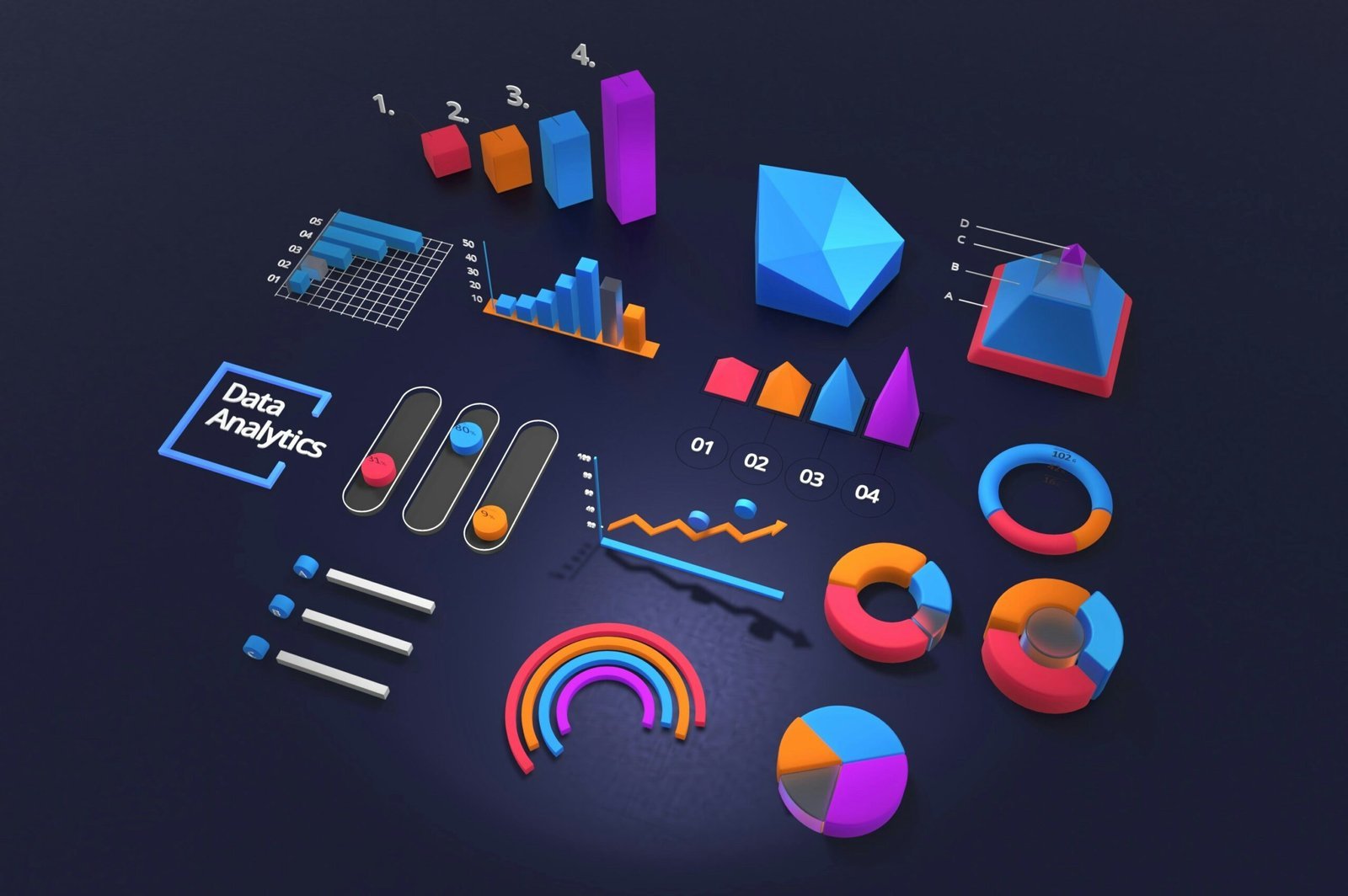
Introduction to AI in Operations Data Analytics
The integration of artificial intelligence (AI) into operations data analytics represents a transformative shift in how organizations manage and interpret their data. At its core, AI encompasses various technologies designed to mimic human intelligence, such as machine learning, natural language processing, and neural networks. These technologies enable systems to learn from data, identify patterns, and make decisions with minimal human intervention.
Machine learning, a subset of AI, further refines this process by allowing systems to improve their performance over time as they are exposed to more data. In the realm of operations data analytics, machine learning algorithms process and analyze vast amounts of data, extracting valuable insights that would be impossible for humans to discern manually. This intersection of AI and data analytics harnesses the strengths of both fields to tackle the increasing volume and complexity of operations data.
Modern enterprises generate enormous quantities of data from varied sources, including IoT devices, customer interactions, supply chain activities, and more. The sheer scale and heterogeneity of this data require advanced analytical tools for effective management and interpretation. AI-driven analytics tools are capable of ingesting diverse datasets, cleaning and processing them, and conducting sophisticated analyses to uncover actionable insights.
Integrating AI into operations data analytics offers several potential benefits. One of the most significant advantages is improved decision-making capability. By leveraging predictive analytics, organizations can foresee future trends, optimize operational processes, and make data-driven decisions that enhance overall efficiency. Additionally, AI can automate routine and repetitive tasks, freeing up human resources to focus on more strategic initiatives.
Another vital benefit is the enhancement of predictive capabilities. AI systems can analyze historical data to predict future outcomes, enabling organizations to anticipate operational issues and adapt their strategies accordingly. This proactive approach not only boosts efficiency but also mitigates risks, ensuring smoother operations.
In conclusion, AI’s integration into operations data analytics marks a critical evolution, equipped to handle the complexities and demands of modern data landscapes, thereby enabling organizations to achieve unprecedented levels of operational excellence.
Current Applications and Case Studies
Artificial Intelligence (AI) has significantly transformed operations data analytics across various industries. In manufacturing, AI-driven predictive maintenance systems are employed to identify potential equipment failures before they occur. This approach not only enhances productivity but also minimizes downtime and reduces maintenance costs. A notable example is General Motors, which has successfully implemented AI to predict and prevent equipment failures, resulting in a 10% increase in plant productivity.
Logistics companies, such as UPS, leverage AI for route optimization and demand forecasting. By analyzing historical data and real-time variables, AI can predict the most efficient delivery routes and optimize load distribution, thereby reducing fuel consumption and operational costs. For instance, UPS’s ORION system has, on average, delivered savings of 10 million gallons of fuel annually, showcasing the substantial benefits of AI integration.
In healthcare, AI-powered analytics are used to streamline operations and enhance patient care. Predictive models can forecast patient admissions, enabling hospitals to better allocate resources. Additionally, AI tools are utilized for optimizing treatment protocols and managing supply chains of critical medical supplies. Mayo Clinic’s adoption of AI for patient management has led to improved operational efficiency and patient outcomes, demonstrating the value of AI in healthcare operations.
Financial institutions have also embraced AI to enhance operational efficiency through advanced data analytics. AI algorithms are used for fraud detection, risk management, and customer service optimization. For example, JPMorgan Chase employs AI to analyze vast datasets for fraud detection, significantly reducing financial losses and enhancing transaction security. Similarly, AI-driven chatbots are widely used for improving customer service efficiency, offering real-time assistance and reducing the burden on human operators.
A range of tools and technologies facilitate these AI applications. Predictive maintenance systems, demand forecasting models, and process optimization solutions are at the forefront, driven by advancements in machine learning and data analytics. These AI applications not only foster greater productivity and cost-efficiency but also pave the way for innovative solutions in operations data analytics.
Challenges and Limitations
While the integration of AI in operations data analytics presents significant opportunities, organizations still face numerous challenges and limitations. First and foremost, the issue of data quality and integration remains a critical obstacle. AI-driven analytics heavily rely on high-quality, well-integrated datasets to generate accurate insights. However, inconsistencies, incomplete datasets, and disparate data sources impede the efficiency and reliability of AI models. To address these concerns, organizations must invest in robust data governance frameworks and advanced data integration tools.
Another significant challenge lies in the complexity of AI models and algorithms. Developing and deploying sophisticated AI models requires advanced expertise in data science, machine learning, and domain-specific knowledge. This necessitates hiring skilled personnel who can design, implement, and maintain these systems, which can be resource-intensive. Additionally, there is a steep learning curve for existing staff members, quickly increasing the demand for specialized training programs to upskill the workforce.
Resistance to change within organizations also proves to be a formidable barrier. The integration of AI into traditional operations workflows can lead to fundamental shifts in how tasks are performed. Employees accustomed to legacy systems may find it difficult to adapt to new AI-driven processes. To mitigate this resistance, organizations need to focus on change management strategies, emphasizing the benefits and efficiencies introduced by AI technologies, and fostering an inclusive culture that embraces innovation.
Ethical and regulatory considerations further complicate the implementation of AI in operations data analytics. Important issues such as data privacy, security, and the ethical use of AI must be meticulously managed. Compliance with regional and international regulations, such as GDPR and HIPAA, adds additional layers of complexity. Organizations are, therefore, compelled to incorporate stringent data protection measures and ethical guidelines to ensure responsible AI usage.
In summing up, although these challenges are substantial, they are not insurmountable. Organizations are investing in advanced technologies and strategic partnerships to improve data quality, simplify AI models, and enhance training programs. By proactively addressing these limitations, the potential for AI in operations data analytics can be fully realized.
Future Trends and Predictions
The future of AI in operations data analytics is poised to be transformative, marked by several emerging trends and technological advancements. One key trend that stands out is the rise of edge computing. By bringing computation and data storage closer to the location where it’s needed, edge computing significantly reduces latency and bandwidth usage. This paradigm shift will empower real-time analytics, enabling organizations to derive immediate insights and make data-driven decisions faster than ever before.
Another pivotal development is the increased adoption of AI-driven automation. Automation, enhanced by AI, promises to streamline routine tasks, reduce human error, and enhance operational efficiency across industries. Organizations will increasingly rely on AI to predict maintenance needs, optimize supply chains, and customize customer experiences in real time. These capabilities will not only improve operational workflows but also foster a new era of proactive business management.
Experts forecast that AI will continue to evolve, becoming more sophisticated and integral to operational strategies. According to a report by Gartner, by 2025, AI will be embedded in nearly every new software product and service. This integration will revolutionize business models, enabling companies to unlock new revenue streams and achieve unprecedented levels of productivity and innovation.
The convergence of AI with other cutting-edge technologies, such as the Internet of Things (IoT) and blockchain, is also anticipated to drive significant advancements in operations data analytics. These technologies will work synergistically to create intelligent, autonomous systems capable of learning, adapting, and scaling without extensive human intervention. For instance, AI-powered IoT devices could enable real-time monitoring and diagnostics of industrial machinery, while blockchain could enhance data security and transparency in supply chain operations.
Envisioning the future, we see a landscape where AI seamlessly integrates into the fabric of daily operations, driving unparalleled efficiency and innovation. Organizations will harness the power of AI to stay competitive, agile, and responsive to ever-changing market demands. This future holds the promise of smarter, more resilient operations, paving the way for groundbreaking advancements in various industries.