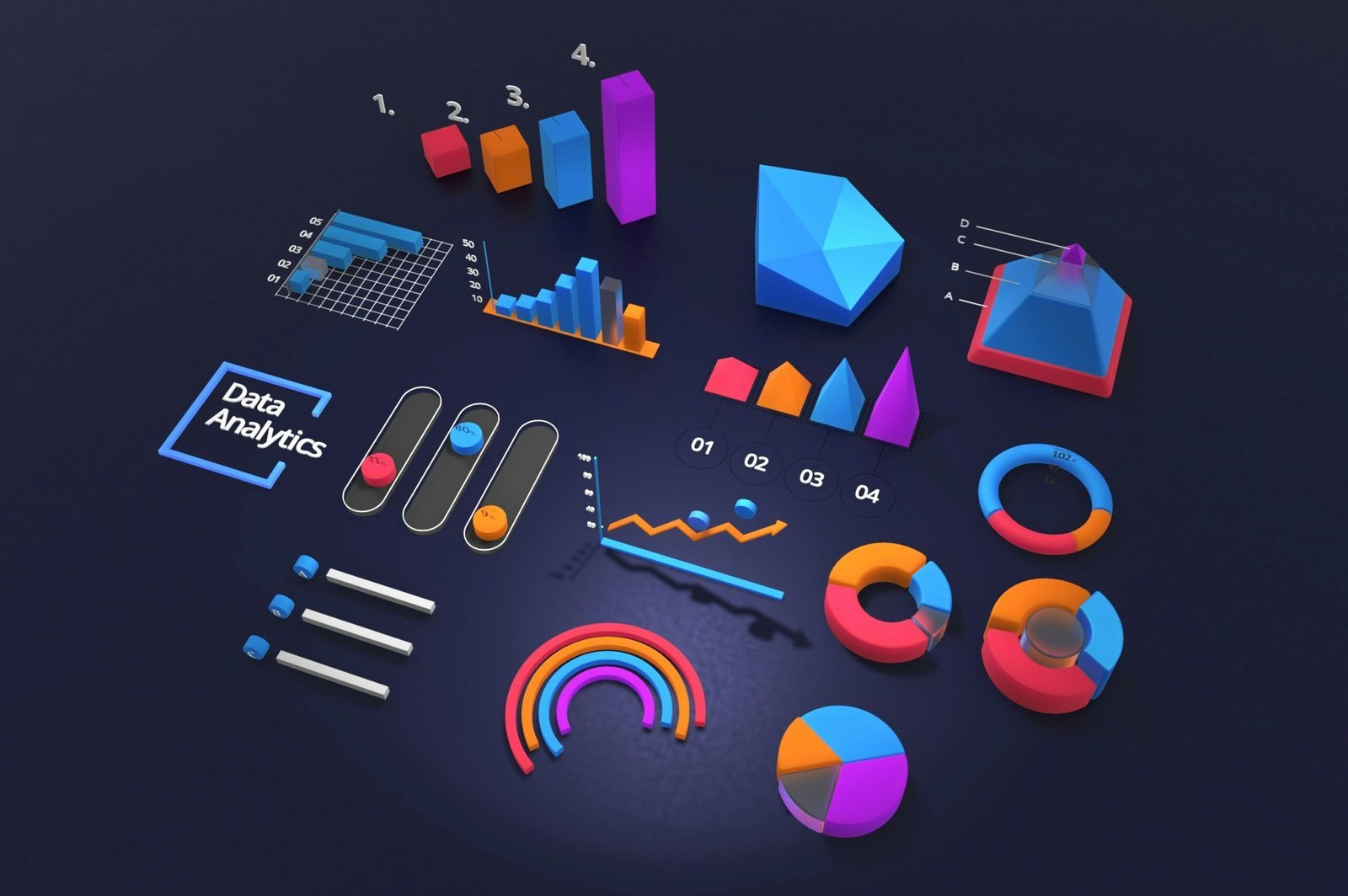
Introduction to Big Data Analytics
Big data analytics represents a transformative approach to processing and interpreting vast amounts of data generated in the digital age. At its core, big data analytics involves the examination of large and complex data sets to uncover hidden patterns, unknown correlations, market trends, and customer preferences. The sheer volume, velocity, and variety of data produced in today’s interconnected world are unprecedented. Organizations across various industries harness these data streams to enhance their decision-making processes, improve operational efficiency, and gain competitive advantages.
The volume of data refers to the massive amounts of information generated every second from numerous sources including social media, sensors, transactions, and more. Velocity pertains to the speed at which new data is generated and processed, necessitating real-time or near-real-time analytics capabilities. The variety dimension encompasses the different types of data available—structured, semi-structured, and unstructured—such as text, images, videos, and log files.
Big data analytics unlocks valuable insights by employing advanced techniques such as machine learning, statistical analysis, and predictive modeling. These insights can drive strategic business decisions, optimize marketing campaigns, enhance customer experiences, and even forecast future trends. For researchers, big data provides an unparalleled opportunity to conduct in-depth studies and achieve breakthroughs in various fields, from healthcare to environmental science.
The power and potential of big data are immense, offering possibilities that were once beyond reach. However, with this great potential comes the necessity for ethical considerations. As organizations and researchers delve deeper into data analytics, they must navigate complex ethical landscapes to ensure that data usage respects individual privacy, maintains transparency, and promotes fairness. This foundation sets the stage to explore the ethical dimensions critical to responsible big data analytics.
The exponential growth of big data analytics has brought to the forefront significant privacy concerns, primarily revolving around the collection and analysis of vast datasets. A critical issue is the potential exposure of Personally Identifiable Information (PII), which includes data points such as names, addresses, social security numbers, and other markers that can directly identify an individual. The aggregation of such information, if mishandled, can lead to serious privacy breaches, ultimately compromising user trust and safety.
One of the fundamental aspects of safeguarding privacy in big data analytics is the implementation of robust data security measures. Data encryption stands out as a pivotal method, as it ensures that information is converted into a secure format that can only be accessed by authorized entities. This process helps to protect sensitive data from unauthorized access during storage and transmission.
Anonymization of data is another critical practice, involving the removal or obfuscation of PII to prevent the identification of individuals within a dataset. Techniques such as data masking, where real data is replaced with fictitious data, and differential privacy, which adds statistical noise to datasets, play a crucial role in maintaining user privacy without compromising the utility of the data for analysis.
Despite these measures, incidences of privacy breaches highlight the risks associated with inadequate security practices. For instance, the 2018 Facebook-Cambridge Analytica scandal exposed the misuse of data from millions of users for political advertising without their consent, raising global awareness about the vulnerabilities in data security protocols. Another notable case is the Equifax data breach in 2017, where sensitive information of approximately 147 million individuals was exposed due to insufficient security measures.
These cases underscore the necessity for organizations to adopt comprehensive data security strategies. Regular security audits, employee training on data handling practices, and adherence to regulatory standards are imperative steps to ensure the protection of user privacy in the realm of big data analytics. As the field continues to evolve, it is paramount for stakeholders to prioritize ethical considerations, fostering an environment where data-driven innovation can thrive without compromising individual privacy rights.
Bias and Fairness in Data Analytics
In the realm of big data analytics, the ethical issues surrounding bias and fairness are of paramount importance. Algorithms, which are the backbone of data analytics, often perpetuate and amplify existing biases present in the data they analyze. These biases can stem from various sources, including historical data, societal stereotypes, and incomplete datasets. When algorithms inherit and propagate these biases, the impact on decision-making can be profound, leading to discriminatory outcomes that affect individuals and communities.
For instance, in the finance industry, biased algorithms can result in unfair lending practices. Historical data reflecting past discrimination against certain demographic groups can lead to higher loan rejection rates for these groups, despite their creditworthiness. In healthcare, biased data can result in unequal treatment recommendations, disproportionately affecting minority groups. Similarly, in law enforcement, predictive policing algorithms trained on biased crime data can unfairly target specific neighborhoods or ethnicities, exacerbating existing disparities.
To mitigate these issues, it is essential to implement strategies that promote fairness and reduce bias in data analytics. One effective approach is to ensure diverse data sourcing. By incorporating data from varied demographics and socioeconomic backgrounds, organizations can minimize the risk of biased analyses. Additionally, regular algorithm audits are crucial. These audits involve systematically reviewing algorithms to identify and address potential biases. They can help in refining the algorithms to ensure they are not perpetuating unfair practices.
Moreover, transparency in algorithm design and decision-making processes can significantly enhance fairness. By openly sharing how algorithms work and the data they rely on, stakeholders can better understand potential biases and work towards mitigating them. Engaging multidisciplinary teams, including ethicists, social scientists, and domain experts, in the algorithm development process can also provide diverse perspectives that help in identifying and addressing biases.
Ultimately, maintaining fairness in data analytics requires a proactive and continuous effort. By recognizing the ethical implications of biased data and implementing strategies to counteract these biases, organizations can foster more equitable decision-making and contribute to a fairer society.
Transparency and Accountability
In the realm of big data analytics, transparency and accountability are paramount for maintaining ethical standards. Organizations must be transparent about their data collection methodologies, storage practices, and usage policies. This transparency builds trust with users and ensures that they are fully informed about how their data is being handled.
The ethical obligation to inform users and obtain their consent for data usage cannot be overstated. Users have a right to know what data is being collected, the purpose behind its collection, and how it will be used. Clear communication and explicit consent are essential to uphold these ethical principles. Failure to do so can undermine user trust and lead to potential ethical and legal ramifications.
Regulations and standards, such as the General Data Protection Regulation (GDPR), play a crucial role in enforcing transparency. The GDPR mandates that organizations must provide clear and accessible information to users about how their data is processed. It also requires that users give explicit consent for data collection and processing activities. These regulations serve as a framework to ensure that organizations are held accountable for their data practices and that user rights are protected.
Algorithmic accountability is another critical aspect of transparency in big data analytics. As decisions increasingly rely on data-driven algorithms, it is essential to have mechanisms in place to address and rectify any wrongful decisions. This requires organizations to regularly audit and evaluate their algorithms to ensure they are fair, unbiased, and do not perpetuate existing inequalities. Transparent documentation of algorithmic processes and decision-making criteria is also necessary to foster trust and accountability.
In conclusion, transparency and accountability in big data practices are foundational to maintaining ethical standards and user trust. By adhering to regulations like the GDPR, informing users, obtaining consent, and ensuring algorithmic accountability, organizations can navigate the complexities of big data analytics ethically and responsibly.