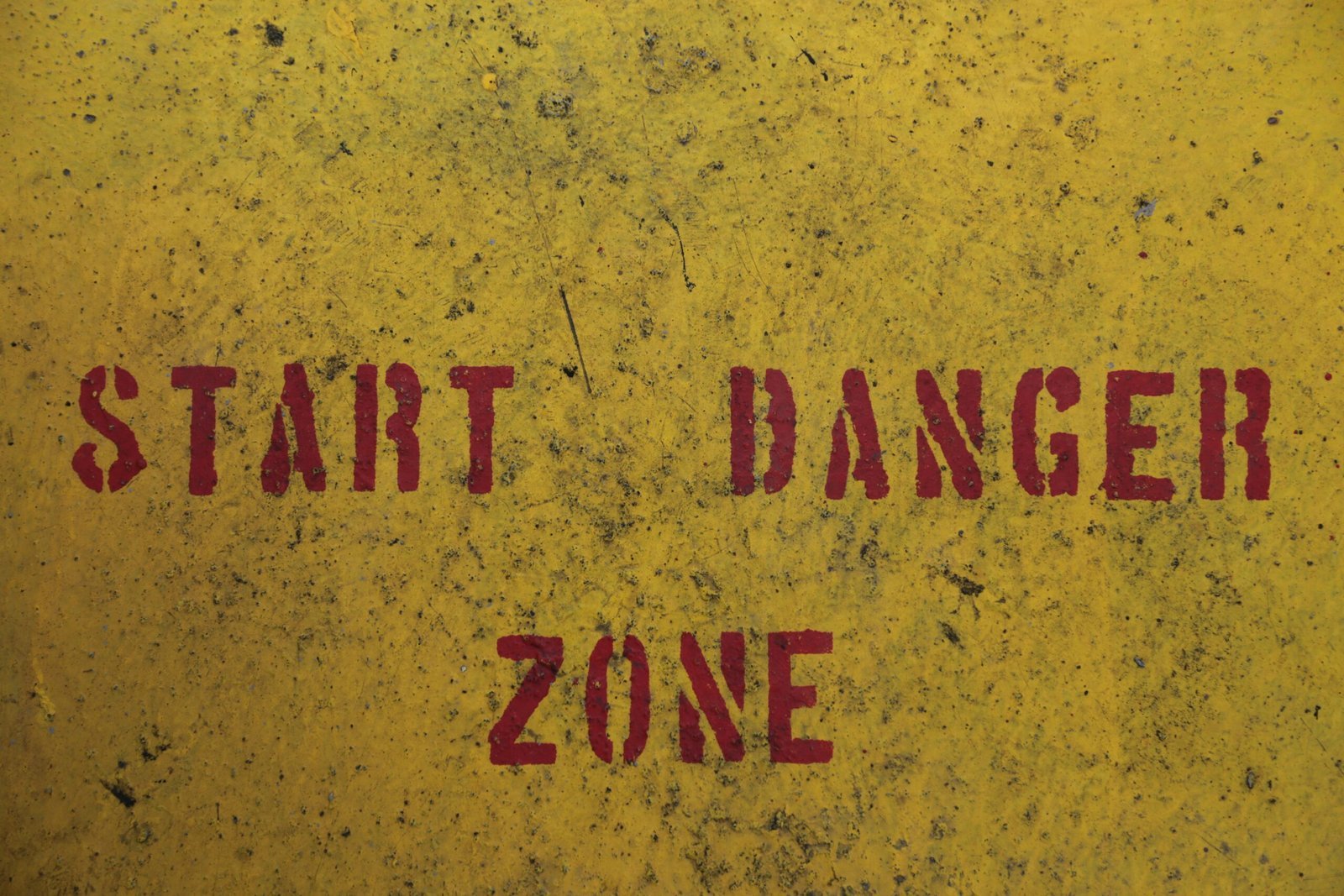
Introduction to AI in Risk Management
In recent years, the role of artificial intelligence (AI) in risk management has gained significant attention across various industries. Traditional methods of risk management, typically reliant on historical data and manual assessment, often fall short in detecting and predicting emerging threats. These conventional practices are time-consuming, prone to human error, and lack the agility required to respond to rapidly evolving risks.
The evolution of AI technologies, however, has introduced transformative capabilities in identifying, assessing, and mitigating risks with high precision and efficiency. By leveraging machine learning algorithms, predictive analytics, and natural language processing, AI systems can analyze vast amounts of data in real-time, uncover patterns, and predict potential risks that might remain undetected by traditional methods.
AI’s role in risk management extends beyond mere data analysis. It facilitates proactive risk mitigation by providing actionable insights, automating decision-making processes, and monitoring risk factors continuously. Industries such as finance, healthcare, cybersecurity, and supply chain management have seen notable improvements in risk management practices through the integration of AI. For instance, in the financial sector, AI helps in detecting fraudulent transactions, forecasting market trends, and managing credit risks with unparalleled accuracy.
The adoption of AI in risk management also marks a shift towards more dynamic, adaptive strategies. Unlike static, rule-based risk management frameworks, AI-driven models can learn from new data, evolving their risk assessment criteria over time. This adaptability ensures that risk management practices remain relevant and effective amid changing market conditions and emerging threats.
As organizations continue to navigate a complex global risk landscape, the need for robust, sophisticated risk management tools becomes ever more critical. AI not only addresses the limitations of traditional risk management methods but also enhances overall preparedness and resilience. This introduction to AI in risk management sets the stage for a deeper exploration into the specific ways AI technologies are revolutionizing how threats are identified and mitigated across different sectors.
How AI Identifies Risks
Artificial Intelligence (AI) has revolutionized risk identification through the application of advanced technologies such as machine learning, natural language processing (NLP), and predictive analytics. These AI technologies are critical in recognizing potential threats across various sectors by efficiently analyzing vast amounts of data, predicting future risks, and uncovering anomalies.
Machine learning, a subset of AI, employs algorithms to learn from historical data and recognize patterns that humans might overlook. These algorithms are pivotal in identifying fraudulent activities in finance, predicting potential health risks in healthcare, and detecting security breaches in cybersecurity. For example, banks utilize machine learning models to analyze transactional data in real-time, pinpointing unusual activities that signal fraudulent behavior.
Natural language processing enables AI systems to understand, interpret, and generate human language. NLP is particularly valuable in identifying risks associated with unstructured data sources, such as emails, social media, and news articles. In healthcare, NLP assists in scrutinizing clinical notes and electronic health records to foresee adverse patient outcomes. Similarly, in the field of cybersecurity, NLP helps in examining threat intelligence reports, allowing for the early identification of potential cyber threats.
Predictive analytics leverages statistical techniques and machine learning models to forecast future events based on historical data. In risk management, predictive analytics aids organizations in anticipating potential risks and devising proactive measures. For instance, insurance companies utilize predictive models to assess the risk profiles of policyholders, ensuring that they adequately price premiums and prepare for any significant claims.
AI’s effectiveness in risk identification heavily depends on the quality of data it processes. High-quality, relevant data sets are essential for training AI models. Poor data quality can lead to inaccurate predictions and overlooked risks. Therefore, ensuring that data is clean, accurate, and comprehensive is critical in maximizing the utility of AI in risk management.
Overall, AI technologies like machine learning, NLP, and predictive analytics considerably enhance the process of identifying risks. By analyzing large datasets in real-time and anticipating potential threats, AI empowers organizations across various sectors, such as finance, healthcare, and cybersecurity, to stay one step ahead of potential risks.
Mitigating Risks with AI
Artificial Intelligence (AI) has become an indispensable tool in risk management, offering advanced strategies and solutions for developing and executing effective risk mitigation plans. AI-driven platforms enable organizations to analyze vast amounts of data to identify potential threats, facilitating the creation of proactive measures. These systems can continuously monitor for anomalies, ensuring that potential risks are identified and addressed before they escalate.
One of the primary advantages of AI in mitigating risks lies in its ability to integrate seamlessly with existing risk management frameworks. By enhancing traditional methods with AI capabilities, organizations can optimize resource allocation and improve decision-making processes. For instance, AI algorithms can prioritize risks based on their potential impact, allowing risk managers to focus their efforts on the most critical areas. Moreover, machine learning models can predict the likelihood of risk events, enabling more effective contingency planning.
Real-world examples highlight the substantial impact of AI on risk mitigation. In the financial sector, for instance, AI is used to detect fraudulent activities by analyzing transaction patterns and identifying deviations from the norm. This proactive approach not only minimizes financial losses but also enhances customer trust. Similarly, in cybersecurity, AI systems can predict and neutralize threats by learning from previous attacks and adapting defense mechanisms accordingly.
Case studies further illustrate AI’s role in improving organizational resilience. For example, a leading manufacturing firm integrated AI into its supply chain management, allowing it to forecast disruptions and optimize inventory levels. This integration resulted in reduced downtime and significant cost savings. In healthcare, AI-driven risk management solutions have been employed to predict patient outcomes and prevent adverse events, thereby improving patient safety and reducing liability for healthcare providers.
In conclusion, AI’s integration into risk management processes significantly enhances an organization’s ability to identify, prioritize, and mitigate risks. By providing more accurate risk assessments and enabling proactive measures, AI not only helps in reducing potential threats but also optimizes resource utilization and strategic decision-making, ultimately bolstering overall organizational resilience.
Challenges and Ethical Considerations
Implementing AI in risk management offers promising opportunities but also presents a series of challenges and ethical considerations that must be meticulously addressed. One of the most significant concerns is data privacy. AI systems require vast amounts of data to function effectively. Ensuring that this data is handled responsibly and securely is paramount. Organizations must comply with stringent data protection regulations, such as GDPR, to safeguard personal information.
Algorithmic bias is another critical issue. AI systems can inadvertently perpetuate or even amplify existing biases present in the data from which they learn. This bias can lead to unfair or discriminatory outcomes, undermining the integrity of risk management processes. It is essential to implement bias detection and mitigation strategies, fostering a more equitable and fair application of AI technologies.
Transparency is vital when deploying AI in risk management. Stakeholders need to understand how AI systems make decisions and assess risks. Transparent AI builds trust and allows for better oversight and accountability. Companies should strive to make their AI models and decision-making processes as transparent as possible, enabling stakeholders to scrutinize and understand AI-driven outcomes.
Over-reliance on AI is another potential pitfall. While AI can augment human capabilities, it is crucial not to diminish the role of human oversight. Dependence on AI without adequate human intervention could lead to oversight of crucial nuances that AI might miss. An effective risk management strategy combines AI’s strengths with human intuition and experience.
To mitigate these challenges, organizations should implement robust governance frameworks. Such frameworks ensure that AI deployments are subject to rigorous ethical standards and accountability measures. Fostering a culture of ethical AI use within the organization is also essential. This involves continuous training, promoting awareness of ethical considerations, and maintaining a vigilant stance towards potential ethical dilemmas.
As we look to the future, the landscape of AI in risk management is bound to evolve. Ongoing advancements in AI technology hold the promise of more sophisticated and nuanced risk assessment capabilities. However, the ethical and practical challenges identified must remain at the forefront of discussions. Ensuring the responsible and ethical use of AI will be key to its successful integration into risk management practices.