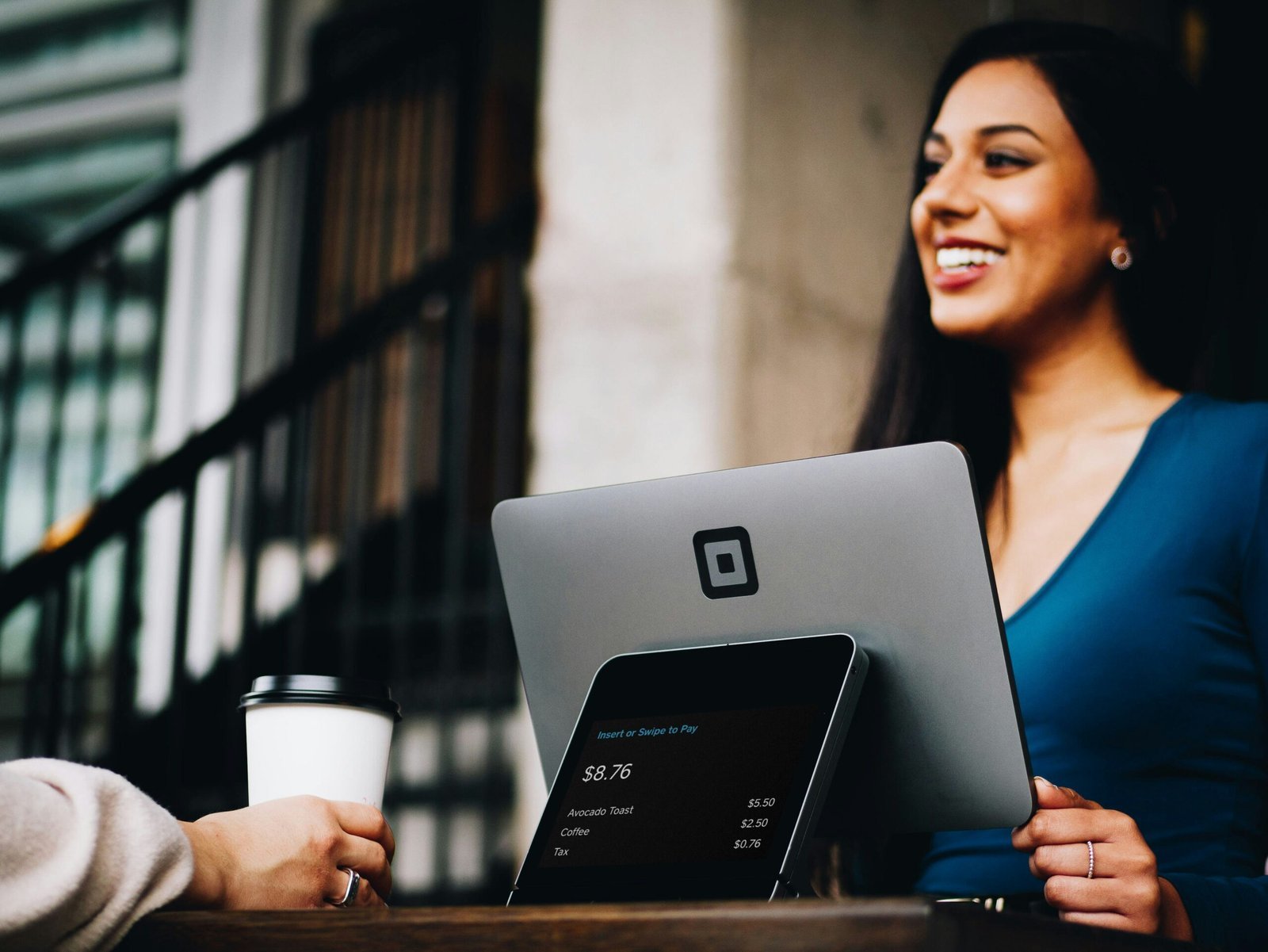
In recent years, artificial intelligence (AI) has revolutionized various aspects of business operations, including customer feedback analysis. Traditional methods of analyzing customer feedback typically involve manual processes which are time-consuming, prone to human error, and incapable of efficiently handling large volumes of data. These manual methods often rely on simple categorization and keyword searches, which do not provide a comprehensive understanding of customer sentiment or emerging trends.
The adoption of AI in customer feedback analysis addresses these limitations by automating the processing and interpretation of feedback data. One of the core advantages of using AI is its ability to handle vast amounts of unstructured data swiftly and accurately. AI technologies can identify complex patterns and correlations that would be impossible to detect manually, thus offering richer and more actionable insights.
Several AI-driven technologies are integral to modern customer feedback analysis. Natural Language Processing (NLP) enables machines to understand and interpret human language, making it possible to analyze textual feedback effectively. This technology goes beyond mere keyword detection, enabling a deeper understanding of context, tone, and sentiment.
Machine Learning (ML), another crucial component, allows systems to improve their analysis models continuously based on new data. ML algorithms can learn from previous feedback, refining their ability to predict and classify customer sentiments over time. This continual learning process ensures that the insights remain relevant and accurate as new data comes in.
Sentiment analysis, a subset of NLP, plays a vital role in discerning the emotional tone behind customer feedback. By categorizing the text as positive, negative, or neutral, sentiment analysis provides a clear picture of customer feelings towards products or services, enabling businesses to make informed decisions.
The integration of these advanced AI technologies offers a more efficient, accurate, and scalable solution for customer feedback analysis. Companies leveraging AI can gain a competitive edge by swiftly translating vast quantities of customer feedback into meaningful, actionable insights, ultimately enhancing customer satisfaction and driving business growth.
Core Technologies in AI-driven Customer Feedback Analysis
Artificial Intelligence (AI) has revolutionized the way businesses analyze customer feedback by employing several advanced technologies. At the core of AI-driven customer feedback analysis are Natural Language Processing (NLP), Machine Learning (ML), and sentiment analysis, each playing a pivotal role in deciphering and interpreting voluminous feedback data.
NLP forms the foundation of AI-enabled customer feedback analysis, facilitating the understanding and interpretation of human language data. By leveraging algorithms to parse and process textual data, NLP allows businesses to handle unstructured feedback from multiple sources such as online reviews, customer surveys, and social media posts. Through techniques like tokenization, lemmatization, and entity recognition, NLP can identify key themes and insights within the feedback that might otherwise be missed.
Machine Learning is another critical component, focusing on predicting trends and patterns derived from customer feedback. ML models can be trained on historical feedback data to uncover correlations and forecast future customer behavior. For instance, supervised learning techniques help in classifying feedback into predefined categories, while unsupervised learning methods can detect hidden patterns without prior labeling. This capability allows businesses to be more proactive in addressing customer needs and improving their products or services.
Sentiment analysis, a specialized branch of NLP, determines the emotions and sentiments conveyed in feedback texts. By assessing the tone and intensity of language used by customers, sentiment analysis helps identify whether the feedback is positive, negative, or neutral. This information is invaluable as it enables companies to gauge customer satisfaction levels accurately. Analyzing sentiments at a granular level can uncover underlying attitudes and drivers behind customer opinions, thus offering deeper insights.
Several real-world applications highlight the successful application of these AI technologies. For instance, a leading e-commerce platform utilized NLP and ML to analyze millions of customer reviews, resulting in a 20% improvement in customer satisfaction through targeted enhancements. Another case involves a global airline using sentiment analysis to monitor social media feedback, enabling them to swiftly address customer grievances and enhance service quality.
Implementing AI for Effective Feedback Analysis
The implementation of AI for customer feedback analysis begins with the systematic collection of feedback data from diverse channels, including surveys, social media, customer reviews, and direct customer interactions. The multi-faceted nature of data sources ensures a comprehensive understanding of customer sentiments and preferences. To maximize data reliability, it is crucial to standardize the format and structure during the collection phase.
Subsequent to data collection, the next critical step involves data cleaning and preprocessing. This stage entails removing any duplicates, null values, and irrelevant information. Techniques such as tokenization, stemming, and lemmatization are employed to prepare textual data, ensuring consistency and readability. The objective of preprocessing is to present a refined dataset that facilitates accurate analysis, thereby mitigating the risk of erroneous outputs.
Selection of suitable AI tools and frameworks is paramount for effective feedback analysis. Options include Natural Language Processing (NLP) platforms like IBM Watson, Google Cloud NLP, and open-source libraries such as TensorFlow and PyTorch. The choice depends on the specific needs and resources of the business, including considerations of scalability, integration capabilities, and cost, among others.
Training AI models constitutes the core of the analysis process. During this phase, historical feedback data is used to train the models, creating predictive algorithms that can identify patterns and trends. It is essential to periodically re-train models with new data to maintain their relevance and accuracy. Techniques such as sentiment analysis, topic modeling, and aspect-based sentiment analysis (ABSA) are typical methodologies employed in this domain.
Businesses should anticipate and proactively navigate potential challenges such as data privacy concerns, model biases, and the requirement for significant computational resources. Ensuring data privacy and compliance with regulations, like GDPR, is non-negotiable. Moreover, implementing bias mitigation strategies is vital to foster fair analysis results.
Ensuring accuracy in AI-driven analysis is an ongoing endeavor. Regular accuracy assessments, error analysis, and model validation are critical practices. Utilizing feedback loops where human experts periodically review the AI outcomes can significantly enhance trust and reliability in the model predictions.
Popular AI tools such as Azure Cognitive Services, Amazon Comprehend, and specialized platforms like MonkeyLearn and Lexalytics provide robust solutions for businesses aiming to harness AI for customer feedback analysis. Adopting these technologies can empower businesses to derive actionable insights and drive customer-centric strategies effectively.
Benefits and Future Trends of AI in Customer Feedback Analysis
The integration of AI in customer feedback analysis offers numerous advantages that can significantly enhance business operations. One of the most prominent benefits is the improvement in customer satisfaction. By leveraging AI technologies, companies can swiftly process vast amounts of feedback data, identifying key patterns and insights that would be labor-intensive for human analysts to discern. This enables quicker response times to customer issues, fostering a more responsive and customer-centric business environment.
Data-driven decision-making is another critical benefit. AI algorithms can analyze feedback data with high precision, providing actionable insights that inform strategic business decisions. These analytics help organizations to understand customer sentiments, preferences, and pain points, facilitating the development of more tailored and effective business strategies. Consequently, companies can create enhanced product and service offerings that better align with customer expectations, driving higher levels of customer engagement and loyalty.
Moreover, incorporating AI into feedback analysis can lead to increased operational efficiency. By automating the data collection and analysis processes, businesses can significantly reduce the time and resources required for manual data handling. This efficiency, in turn, permits staff to focus on more value-added activities, such as crafting personalized customer experiences and innovating new solutions based on AI-driven insights.
Looking ahead, the future trends in AI for customer feedback analysis promise to revolutionize the landscape further. Advancements in natural language processing (NLP) and machine learning are expected to enhance the accuracy and depth of sentiment analysis, offering a more nuanced understanding of customer emotions and opinions. Integration with other business systems, such as CRM and marketing automation platforms, will enable even more seamless and comprehensive feedback loops, driving a unified approach to customer relationship management.
Businesses need to stay ahead of these developments by continuously investing in AI technologies and upskilling their workforce to harness the full potential of AI-driven feedback analysis. Embracing these future trends will not only position companies at the forefront of innovation but also ensure they remain competitive in an increasingly customer-centric market.