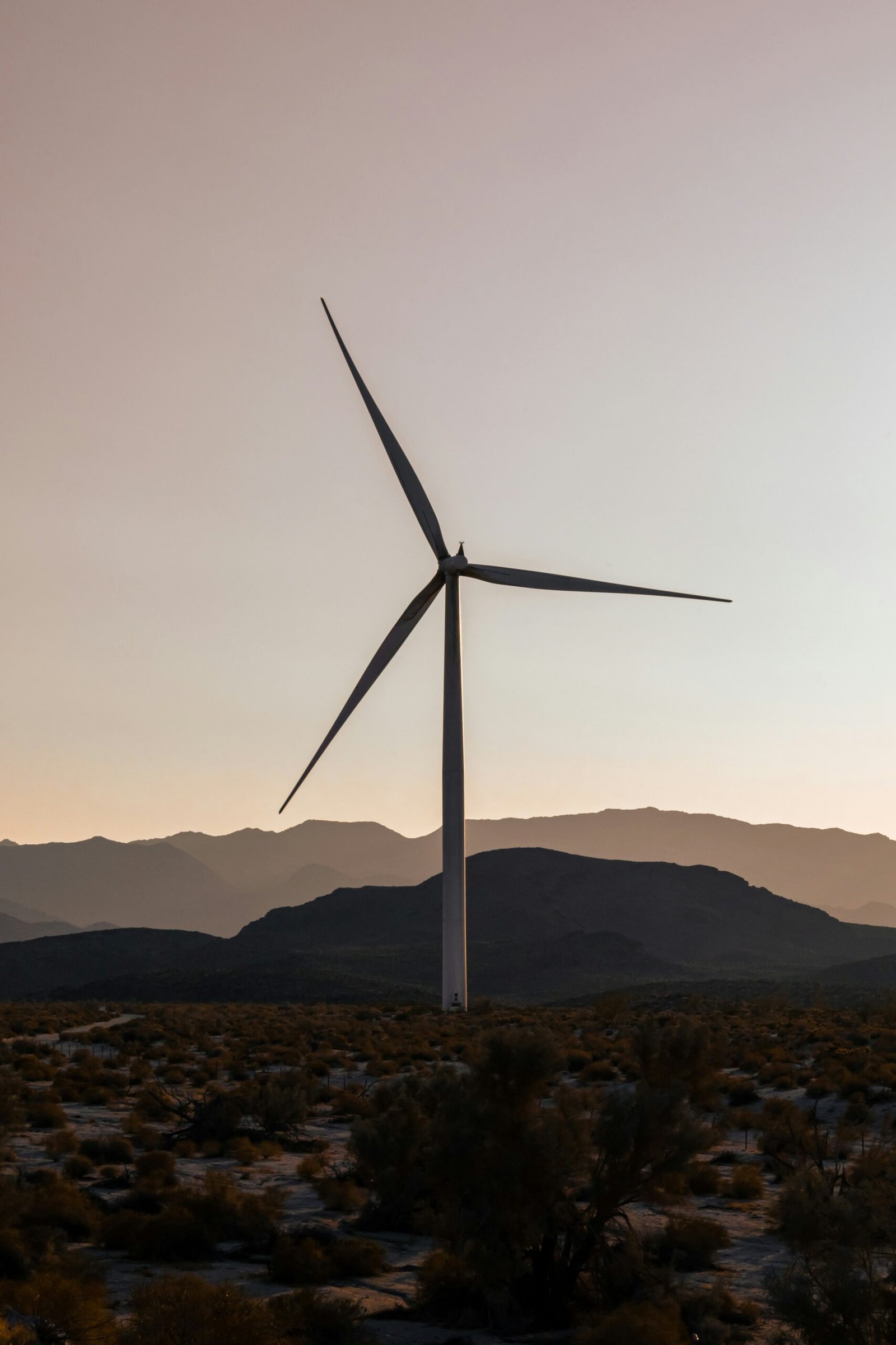
Introduction to AI in Renewable Energy
The intersection of artificial intelligence (AI) and renewable energy management represents a pivotal advancement in the quest for sustainable energy solutions. AI encompasses a range of technologies, including machine learning, neural networks, and data analytics, each playing a crucial role in optimizing energy systems. At its core, AI enables machines to learn from data, identify patterns, and make decisions with minimal human intervention. Machine learning algorithms can, for instance, predict energy consumption patterns, while neural networks can model complex systems and improve energy forecasts. Data analytics aids in interpreting vast amounts of information generated by renewable energy sources, transforming raw data into actionable insights.
One of the primary reasons for the increased adoption of AI in renewable energy management is its ability to enhance efficiency and reliability. Renewable sources, such as solar, wind, and hydroelectric power, are inherently variable and dependent on environmental conditions. AI systems can analyze weather patterns, predict energy generation, and adjust the energy grid accordingly, thus ensuring a stable supply of power. These technologies also optimize energy storage and distribution, reducing wastage and aligning supply with demand in real-time.
The urgency of integrating renewable energy sources has never been greater, driven by the pressing challenge of climate change and the implementation of stringent government policies aimed at reducing carbon emissions. Nations across the globe are setting ambitious targets for renewable energy adoption, and AI stands out as a key enabler in achieving these goals. By streamlining operations, reducing costs, and improving the predictability of renewable energy systems, AI not only supports the transition to cleaner energy but also makes it economically viable.
In summary, the fusion of AI with renewable energy management heralds a new era of innovation. By leveraging advancements in machine learning, neural networks, and data analytics, AI offers a sophisticated toolkit for addressing the complexities of renewable energy systems. This synergy is essential for optimizing the performance and sustainability of energy grids, ultimately contributing to a more resilient and eco-friendly energy landscape.
Artificial Intelligence (AI) has significantly transformed the landscape of energy forecasting, particularly in the realm of renewable energy management. By leveraging machine learning algorithms, AI systems can analyze vast arrays of historical weather data, energy consumption patterns, and other critical variables to predict future energy demands with remarkable accuracy. This advanced forecasting capability is particularly crucial for managing intermittent renewable energy sources like wind and solar power, which are heavily influenced by weather conditions.
Machine learning models utilize historical data sets, encompassing everything from temperature fluctuations to cloud cover and wind speeds, to generate predictions about future energy production and consumption. For example, by examining past weather trends and current meteorological data, AI systems can forecast how much solar power a photovoltaic farm is likely to generate in the coming week. Similarly, wind farm operators can use predictive models to estimate wind speeds and, consequently, the potential energy output from their turbines.
Numerous real-world applications showcase the effectiveness of AI-driven energy forecasting. In Spain, for instance, the electricity system operator Red Eléctrica de España uses AI to anticipate electricity demand and renewable energy supply. By integrating forecasts into their grid management protocols, they can optimize energy distribution, reduce reliance on fossil fuels, and minimize costs. Similarly, companies like IBM and Google have developed sophisticated AI platforms that incorporate energy demand forecasting into their operational frameworks, enhancing the stability and efficiency of renewable energy systems.
Another notable example is the collaboration between the U.S. Department of Energy and various AI research institutes to develop advanced forecasting models for wind and solar energy. These models help grid operators make informed decisions about energy storage and distribution, ensuring that renewable energy is utilized to its fullest potential.
In conclusion, AI-driven energy forecasting is a pivotal element in modern renewable energy management. By offering precise predictions on energy production and consumption, AI not only enhances the reliability of renewable energy sources but also facilitates their integration into the broader energy grid, ultimately fostering a more sustainable and efficient energy ecosystem.
Artificial intelligence (AI) has become a crucial asset in the optimization of energy production and distribution, especially in managing renewable energy resources. Renewable energy sources such as solar and wind are inherently variable and intermittent. These fluctuations pose challenges to maintaining a stable and reliable energy supply. AI has stepped in to address these challenges by leveraging advanced algorithms and real-time data analysis.
Managing Variability and Intermittency
AI systems help balance the supply and demand of renewable energy by predicting energy production patterns and adjusting outputs dynamically. For instance, predictive models can forecast the performance of solar panels based on weather conditions and historical data. Such insights enable AI systems to maximize energy production during peak sunlight hours while minimizing the impact of cloudy periods. Similarly, machine learning algorithms can predict wind patterns, allowing wind turbines to adjust blade angles for optimal energy capture.
Real-time Balancing
One of the most significant benefits of incorporating AI in renewable energy management is real-time balancing of energy supply and demand. AI-powered smart grids utilize sensors and data analytics to monitor energy consumption and generation continuously. These systems can reroute energy flows to where it is most needed, thereby reducing wastage and maintaining stability. For example, during periods of low demand, excess energy can be redirected to storage systems or shared with neighboring grids.
Smart Cities and Microgrids
Successful deployments of AI in renewable energy management can be seen in smart cities and microgrid systems. Cities like Singapore and Amsterdam have implemented AI-driven solutions that optimize energy production and distribution across urban landscapes. Microgrids, smaller-scale electricity networks, often operate independently or in conjunction with larger grid systems. AI can enhance the efficiency of these microgrids by dynamically managing energy sources and storage, ensuring sustainable and reliable electricity supply at the local level.
AI’s role in renewable energy management is continually evolving. Ongoing advancements in technology promise to further enhance the efficiency, reliability, and sustainability of renewable energy systems. As AI continues to advance, its integration into energy production and distribution will undoubtedly play a pivotal role in shaping the future of sustainable energy.
Future Trends and Challenges
As we look to the future, the integration of AI in renewable energy management promises to reshape the energy landscape. Emerging trends suggest significant advancements in AI algorithms, which will enhance the efficiency and predictive capabilities of energy management systems. For instance, machine learning models are becoming increasingly sophisticated in forecasting energy production from renewable sources such as wind and solar power, leading to more accurate and reliable energy supply predictions.
The proliferation of Internet of Things (IoT) devices further amplifies the role of AI in this domain. IoT sensors can collect real-time data on various parameters, including weather patterns, energy consumption, and equipment performance. This data, when processed by AI systems, can optimize energy distribution, reduce waste, and drive down operational costs. The synergistic relationship between AI and IoT will likely become a cornerstone of advanced energy management systems.
However, the deployment of AI in renewable energy is not without its challenges. One significant concern is data privacy. As IoT devices gather vast amounts of sensitive information, ensuring the confidentiality and integrity of this data becomes paramount. Robust cybersecurity measures must be implemented to protect against potential breaches that could jeopardize both individual privacy and the stability of energy grids.
Ethical considerations also play a crucial role in the integration of AI systems. There is an ongoing debate about the extent to which AI should be autonomous in making critical decisions that affect energy distribution and consumption. It is essential to establish ethical guidelines to prevent misuse and ensure that AI applications align with broader societal values.
Finally, the evolving regulatory landscape will be pivotal in overseeing AI integration in the energy sector. Policymakers need to develop comprehensive frameworks that address the unique challenges posed by AI technologies. These regulations should promote innovation while safeguarding public interest, ensuring that AI contributes positively to the advancement of renewable energy management.