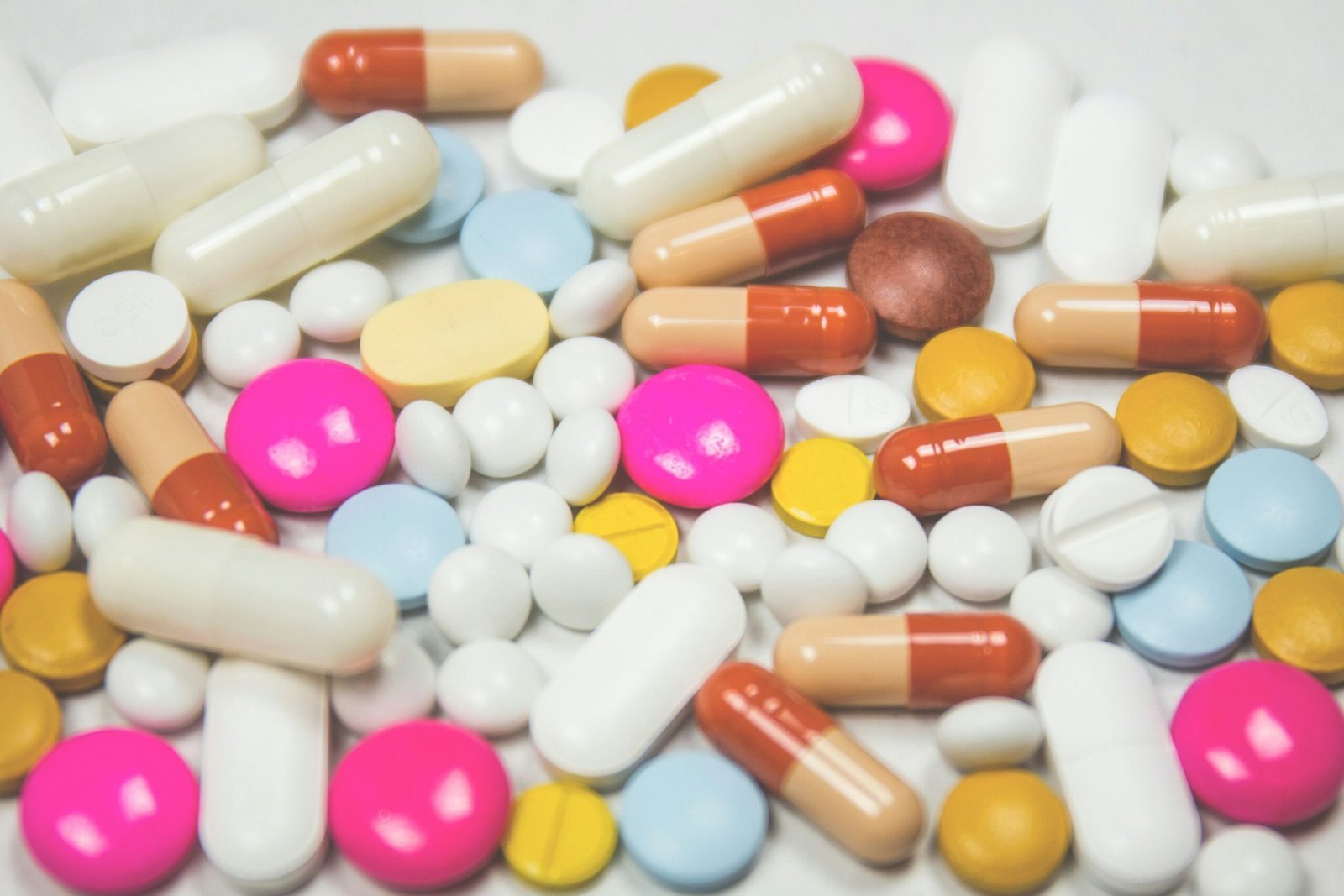
Introduction to Digital Twins in Healthcare
Digital twins are an emerging technology in the healthcare sector, playing a pivotal role in personalizing medicine and enhancing treatment outcomes. At its core, a digital twin is a virtual replica of a physical entity or system. This advanced technology allows intricate modeling and simulation of various scenarios, thereby offering invaluable insights and predictive analysis.
In the context of healthcare, digital twins are utilized to create personalized models of patients. These models integrate diverse data streams, including genetic information, medical histories, and lifestyle factors, to form comprehensive, real-time digital replicas of patients. This consequential amalgamation of data enables healthcare providers to visualize and predict the potential impact of treatments, leading to more precise and tailored medical interventions.
The significance of digital twins in healthcare cannot be overstated. By providing a holistic and dynamic representation of patient health, digital twins facilitate the customization of treatment plans, thereby optimizing patient outcomes. They allow for the safe and effective testing of medical procedures and interventions in a virtual environment before actual clinical application.
Moreover, digital twins enable continuous monitoring and updating of patient models as new data becomes available. This adaptability is critical in managing chronic diseases and conditions that require ongoing treatment adjustments. As a result, digital twins play a fundamental role in advancing precision medicine, reducing healthcare costs, and improving overall patient care quality.
Benefits and Applications of Digital Twins in Personalized Medicine
Digital twins, defined as virtual replicas of physical entities, are transforming the landscape of personalized medicine. By creating digital twins of patients, healthcare providers can simulate various treatment scenarios, predict individual responses, and customize healthcare interventions. These digital representations offer unparalleled insights that could revolutionize patient care.
One of the foremost benefits of digital twins in personalized medicine is their ability to simulate different treatment options without any risk to the patient. For example, by modeling a patient’s cardiovascular system, a digital twin can help cardiologists test the effectiveness of different medication dosages or surgical interventions. This predictive capability ensures that patients receive the most effective treatments tailored specifically to their unique physiology.
Moreover, digital twins enable a more proactive approach to healthcare. By continuously updating the model with real-time data, healthcare providers can predict potential health issues before they manifest. For instance, a digital twin can help monitor a diabetic patient’s glucose levels and predict spikes, allowing for timely adjustments in treatment plans. Such proactive interventions can enhance patient outcomes significantly, reducing hospital readmissions and healthcare costs.
Successful implementations of digital twins already illustrate their potential. A notable case is the use of digital twins in oncology. By creating a digital twin of a tumor, oncologists can simulate various treatment responses, optimizing chemotherapy regimens to maximize efficacy while minimizing side effects. Another example is in orthopedic surgery, where digital twins of bones and joints guide surgical planning and postoperative rehabilitation, ensuring better patient recovery.
Digital twins also hold promise in drug development and precision therapies. By modeling human physiology with exceptional accuracy, they facilitate the testing of new drugs on virtual patients, accelerating the drug discovery process. This approach not only reduces the time and cost associated with clinical trials but also increases the likelihood of developing patient-specific treatments that are more effective and have fewer side effects.
Indeed, as technology advances, the integration of digital twins in personalized medicine is set to grow. Their ability to provide individualized simulations and predictive analytics makes them indispensable tools in the quest for more personalized, effective, and efficient healthcare solutions.
Challenges and Limitations of Implementing Digital Twins
The integration of digital twins in healthcare, while promising, is not without its challenges. One of the foremost concerns is the high cost and complexity involved in developing and maintaining digital twin models. These models require vast amounts of data and sophisticated algorithms, necessitating significant investment in both technology and expertise. The financial burden can be especially prohibitive for smaller healthcare providers and institutions, making widespread adoption challenging.
Data privacy and security form another critical challenge. Digital twins rely heavily on patient data, which includes sensitive personal and medical information. Ensuring this data is protected against breaches and unauthorized access is paramount. This necessitates robust cybersecurity measures which, although effective, can add to the overall complexity and cost of digital twin technologies. Furthermore, addressing concerns related to patient consent and data ownership remains essential to maintaining trust and adherence to ethical standards.
Technological barriers also pose significant hurdles. The development of accurate digital twin models requires precise and comprehensive datasets, which are not always readily available. The integration of these models with existing healthcare systems can be fraught with compatibility issues, and the need for real-time data processing can further complicate implementation. Moreover, the sophistication required in simulating highly complex biological systems is challenging, often leading to potential inaccuracies in the models.
Regulatory and ethical considerations add another layer of complexity. The use of digital twins in patient care must comply with stringent regulations to ensure patient safety and efficacy. Regulatory bodies demand rigorous validation and testing of these models to prevent any adverse outcomes. Ethical considerations, such as the potential for digital twins to inadvertently exacerbate healthcare inequities, must be continually addressed through inclusive and transparent policymaking.
Ultimately, ensuring the accuracy and reliability of digital twins necessitates arduous validation processes. These models must be continually tested and updated against real-world outcomes to maintain their utility in patient care. This iterative process requires continual collaboration across multiple disciplines, adding to the already substantial resource requirements.
Future Prospects and Innovations in Digital Twin Technology
As we look forward, the potential of digital twin technology in healthcare is both exciting and expansive. The continual advancements in artificial intelligence (AI), machine learning, and big data analytics are poised to significantly enhance the capabilities of digital twins. By leveraging AI, digital twins can offer predictive analytics and real-time decision-making support, thus enabling a more proactive approach to patient care. Machine learning algorithms can process vast amounts of patient data to identify patterns and predict health outcomes with greater accuracy. In this context, digital twins not only replicate physical conditions but also adapt and evolve with new data, becoming more personalized and precise over time.
Emerging trends point to the integration of digital twin technology with the Internet of Medical Things (IoMT) and telehealth. The IoMT’s expansive network of connected devices—ranging from wearable sensors to smart diagnostic tools—can continuously feed real-time data into digital twin models. This continuous flow of information ensures that digital twins remain up-to-date and reflective of the patient’s current health status. Furthermore, telehealth platforms can utilize digital twin data to deliver remote consultations that are deeply personalized, potentially revolutionizing access to healthcare by making expert opinions and personalized treatment plans widely available.
The broader implications for healthcare systems are profound. Digital twins hold the promise of democratizing personalized medicine by making tailored healthcare accessible to a broader population. This could reduce the burden on healthcare facilities by preemptively managing conditions and reducing hospital admissions through more effective outpatient care. Additionally, digital twin technology can aid in the development of new treatments and drugs by providing detailed simulation models for testing and validation, thereby accelerating innovation in medical research.
With such advancements on the horizon, the integration of digital twin technology into mainstream healthcare seems not only possible but inevitable. As digital twins become more sophisticated and their applications more diverse, the landscape of patient care and medical research is set to transform, heralding a new era of personalized, efficient, and accessible healthcare.