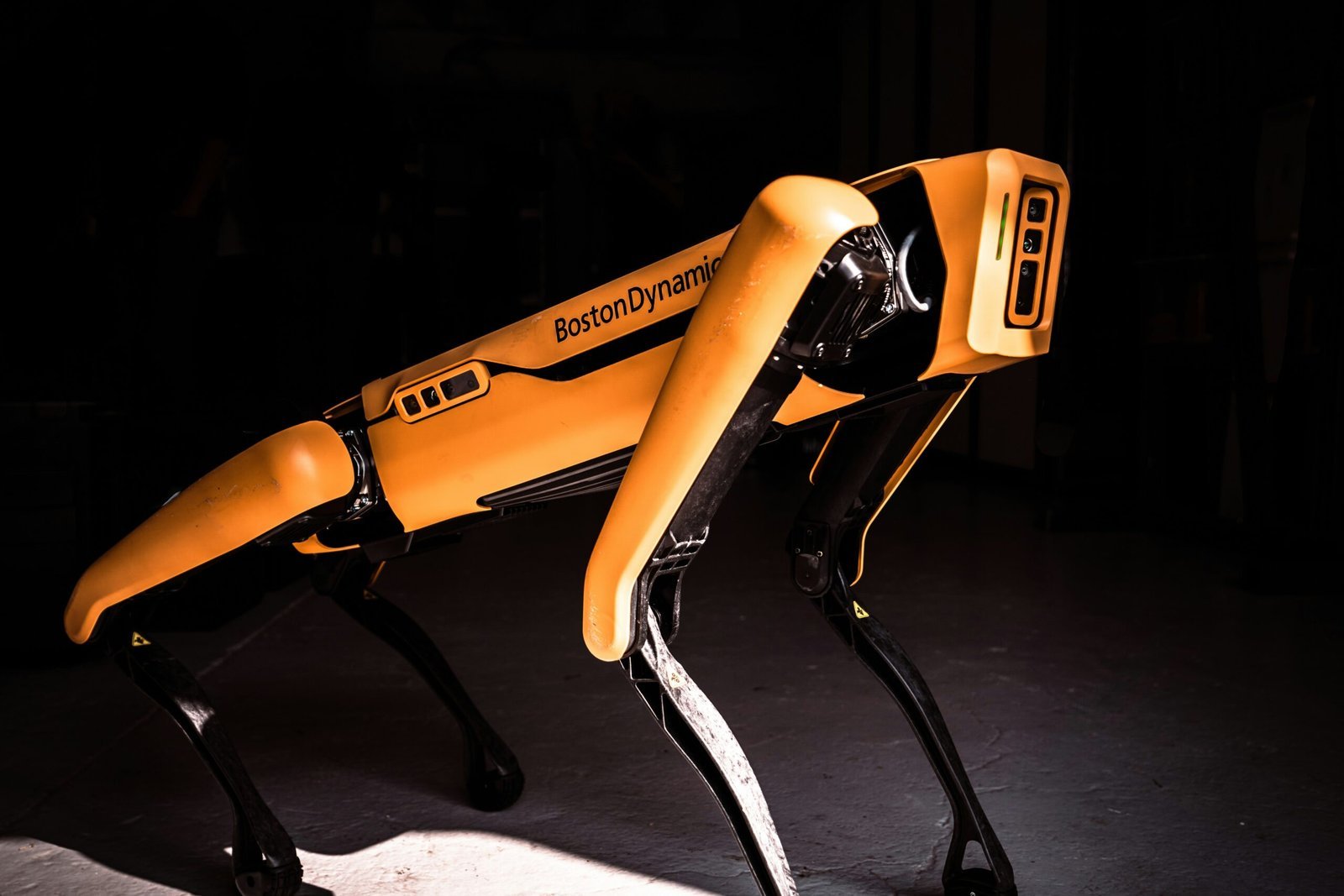
Introduction to Predictive Maintenance
Predictive maintenance is an innovative approach that aims to anticipate equipment failures before they occur, thus significantly reducing unplanned downtime and maintenance costs. Unlike traditional maintenance strategies, predictive maintenance leverages advanced data analytics and machine learning algorithms to predict when a failure might happen. This proactive approach stands in stark contrast to reactive maintenance, which responds to equipment failures after they have occurred, often resulting in costly downtime and repairs.
The limitations of preventive maintenance also highlight the importance of predictive maintenance. Preventive maintenance involves regularly scheduled inspections and repairs, which, while reducing unexpected breakdowns, can still be inefficient. Equipment might undergo maintenance that isn’t necessary, leading to increased operational costs and extended downtime. Predictive maintenance addresses these inefficiencies by utilizing real-time data from sensors and other monitoring devices to assess the actual condition of equipment and predict potential failures.
Data plays a crucial role in the effectiveness of predictive maintenance strategies. Sensors and IoT devices collect vast amounts of data on various parameters such as temperature, vibration, and pressure. This data is then processed and analyzed using machine learning algorithms to identify patterns and anomalies that precede equipment failures. By understanding these patterns, organizations can take timely actions to repair or replace parts before a malfunction occurs.
The implementation of predictive maintenance not only enhances operational efficiency but also extends the lifespan of machinery and equipment. It allows industries such as manufacturing, energy, and transportation to optimize their maintenance schedules, reduce costs, and improve overall productivity. Through the integration of machine learning and advanced analytics, predictive maintenance transforms how businesses manage their maintenance operations, ensuring higher reliability and performance of their assets.
The Role of Machine Learning in Predictive Maintenance
Machine learning technologies are revolutionizing predictive maintenance by enabling more precise and proactive strategies for equipment management. Key techniques, such as supervised learning, unsupervised learning, and reinforcement learning, play pivotal roles in this transformation.
Supervised learning, one of the most widely used approaches, involves training algorithms on labeled datasets where the outputs are known. This technique can predict equipment failures by analyzing historical maintenance records, sensor data, and operational conditions. For example, a supervised learning model could be trained on historical data from manufacturing machines to predict when a component is likely to fail, allowing maintenance teams to schedule repairs before a breakdown occurs.
Unsupervised learning, on the other hand, deals with unlabeled data and is used to identify patterns and anomalies that may not be immediately apparent. This technique is particularly useful for detecting unusual behavior in machinery that could indicate impending failure. For instance, clustering algorithms can group similar operating states and highlight outliers, thus enabling early detection of potential issues.
Reinforcement learning is another powerful technique where algorithms learn to make decisions by trial and error, receiving feedback from their actions. This method can optimize maintenance schedules by continuously learning the most cost-effective and least disruptive times to perform maintenance tasks based on real-time data and operational constraints.
The benefits of incorporating machine learning into predictive maintenance are substantial. One significant advantage is the increased accuracy of failure predictions, which can drastically reduce unplanned downtime and maintenance costs. Machine learning algorithms are also capable of processing vast amounts of data from multiple sources, providing a comprehensive analysis that human operators would find challenging to achieve manually. Furthermore, these technologies can adapt and improve over time, continually enhancing the reliability and efficiency of maintenance processes.
In summary, the integration of machine learning in predictive maintenance offers a robust framework for identifying potential failures before they occur, thereby optimizing maintenance operations and ensuring the longevity and reliability of equipment.
Implementation Strategies and Challenges
Implementing a machine learning-based predictive maintenance system is a multifaceted process that requires meticulous planning and execution. The journey begins with data collection. High-quality sensor data, gathered through IoT devices, is paramount. These sensors monitor machinery conditions and collect pertinent data such as temperature, vibration, and pressure. The data must be comprehensive and continuous to predict potential failures accurately.
Following data collection, data preprocessing is a critical step. Raw data often contains noise, missing values, and inconsistencies that can adversely affect model performance. Techniques such as data cleaning, normalization, and transformation are employed to prepare the data for analysis. Ensuring data quality at this stage is essential for developing reliable predictive models.
The next step involves model selection. Depending on the nature of the machinery and the type of failures to be predicted, various machine learning algorithms such as regression models, neural networks, or decision trees may be appropriate. The choice of the model significantly impacts the predictive maintenance system’s efficacy.
Once a suitable model is selected, the process of model training begins. This involves feeding the preprocessed data into the model and adjusting it to learn patterns that signify impending equipment failures. It’s critical to validate the model using historical data to ensure its accuracy and reliability.
After successful training, the model is ready for deployment. Integration with existing systems is a significant challenge at this stage. The predictive maintenance system must seamlessly work within the existing IT infrastructure, requiring close collaboration between data scientists and IT professionals.
Despite the systematic approach, several challenges must be addressed. Data quality issues can impede the model’s performance. Ensuring high-quality data through regular sensor maintenance and calibration is vital. Another challenge is the need for domain expertise. Collaboration with industry experts is crucial to understanding the specific failure modes and maintenance needs of the machinery.
Finally, integration with existing systems can be complex due to legacy systems and outdated technologies. Ensuring compatibility and smooth communication between the predictive maintenance system and existing IT infrastructure is essential. By addressing these challenges diligently, organizations can successfully implement a machine learning-based predictive maintenance system, ultimately enhancing operational efficiency and reducing downtime.
Case Studies and Future Trends
Several real-world case studies exemplify the transformative potential of machine learning in predictive maintenance across various sectors. In the manufacturing industry, General Motors (GM) has leveraged machine learning algorithms to enhance the uptime and efficiency of its machinery. The implementation of predictive maintenance at GM’s facilities led to a significant reduction in unexpected machine failures, translating to a 50% decrease in downtime and a 20% increase in overall production efficiency. By analyzing sensor data and historical maintenance records, GM’s machine learning models can predict potential equipment failures well in advance, allowing for timely interventions.
Similarly, in the energy sector, Siemens has deployed machine learning for predictive maintenance in its wind turbines. Siemens uses advanced analytics to monitor and predict the performance of turbine components, enabling proactive maintenance scheduling. This approach has resulted in a 30% reduction in maintenance costs and a 15% increase in the turbines’ operational efficiency. By minimizing unplanned outages and optimizing maintenance activities, Siemens has managed to significantly enhance the reliability and sustainability of its energy production.
The transportation industry has also witnessed noteworthy successes with predictive maintenance. The Metropolitan Transportation Authority (MTA) in New York City utilizes machine learning to monitor the health of its subway cars. By analyzing vibration patterns and other sensor data, MTA’s predictive maintenance system can forecast mechanical issues before they escalate. This has led to a 40% reduction in service disruptions and improved overall commuter experience by ensuring more reliable train services.
Future Trends in Predictive Maintenance
Looking ahead, several emerging trends are poised to reshape the landscape of predictive maintenance. The integration of artificial intelligence (AI) and machine learning algorithms continues to advance, driving more accurate and sophisticated predictive models. These enhancements enable more precise failure predictions and tailored maintenance schedules, further optimizing operational efficiency across various industries.
Edge computing is another emerging trend with significant implications for predictive maintenance. By processing data closer to the source, edge computing minimizes latency and allows for real-time decision-making. This is particularly advantageous for industries where immediate responses to equipment health are critical, such as aerospace and defense. The combination of edge computing and machine learning paves the way for more responsive and resilient predictive maintenance solutions.
Moreover, the concept of Predictive Maintenance as a Service (PMaaS) is gaining traction. PMaaS offers companies the ability to outsource their predictive maintenance needs to specialized service providers. This model provides access to cutting-edge technology and expertise without the need for significant in-house investment. As a result, even smaller enterprises can reap the benefits of predictive maintenance, driving broader adoption across diverse sectors.
In conclusion, the future of predictive maintenance powered by machine learning holds immense promise. With continuous advancements in AI, edge computing, and service models like PMaaS, companies across various industries can anticipate unprecedented levels of efficiency, reliability, and cost-effectiveness in their maintenance operations.